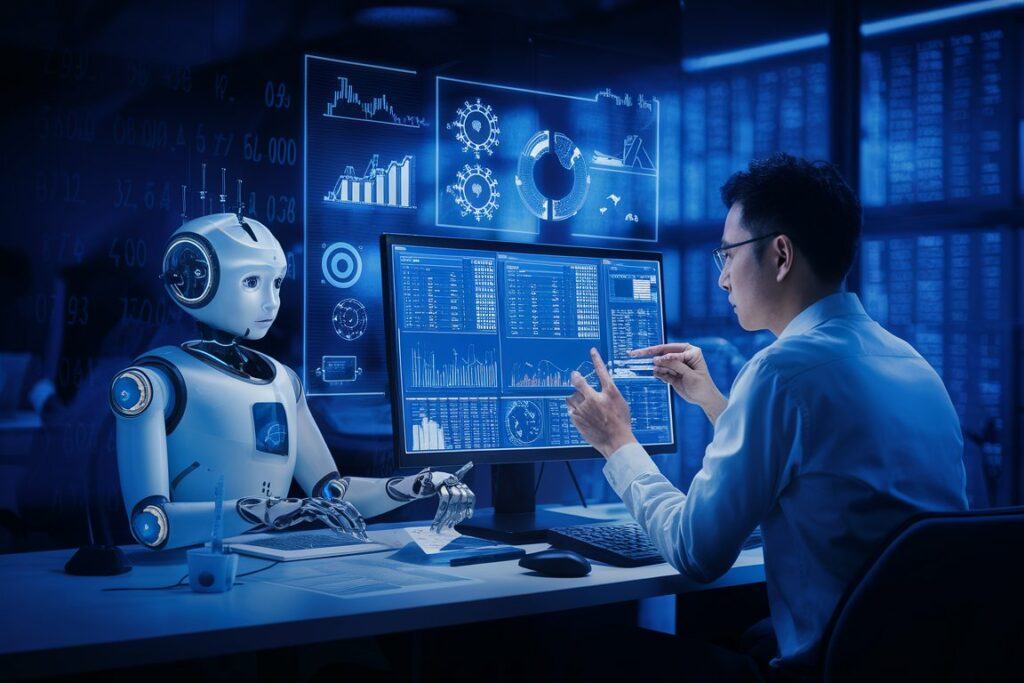
In today’s dynamic financial landscape, the integration of artificial intelligence (AI) has revolutionized risk management practices within the banking sector.
“Navigating Complexity: AI Strategies for Enhanced Risk Mitigation in Banking”
Financial analysts are leveraging AI technologies to enhance decision-making processes, mitigate risks, and optimize operational efficiency like never before.
Explore How AI Enhances Decision-Making and Mitigates Risks in Finance
Understanding AI in Risk Management
AI in risk management involves the application of machine learning algorithms and predictive analytics to assess, predict, and mitigate potential financial risks. Traditionally, risk assessment relied heavily on historical data and statistical models.
However, AI introduces a paradigm shift by processing vast amounts of data at unprecedented speeds, identifying patterns, and generating insights that enable proactive risk management strategies.
Key Applications of AI in Risk Management
1. Predictive Analytics: AI algorithms analyze historical data to identify trends and patterns that may indicate future risks. By leveraging predictive analytics, financial analysts can anticipate market fluctuations, credit defaults, and other potential threats, enabling preemptive actions to mitigate adverse impacts.
2. Natural Language Processing (NLP): NLP enables the analysis of unstructured data such as news articles, social media feeds, and regulatory filings. By extracting valuable insights from textual data, financial analysts can gauge market sentiment, regulatory changes, and emerging risks in real-time.
3. Algorithmic Trading: AI-powered trading algorithms execute transactions based on predefined criteria and market conditions. These algorithms can react swiftly to market movements, optimize portfolio performance, and manage risks associated with high-frequency trading.
4. Fraud Detection: AI algorithms detect unusual patterns in transaction data to flag potential fraudulent activities promptly. By continuously learning from new data, these systems adapt to evolving fraud schemes, providing robust protection against financial crimes.
5. Credit Risk Assessment: AI enhances credit risk assessment by analyzing borrowers’ financial histories, market conditions, and other relevant factors. This enables financial institutions to make informed lending decisions while minimizing default risks.
Benefits of AI in Risk Management
1. Enhanced Accuracy and Efficiency: AI algorithms process vast datasets with high accuracy, reducing the margin for human error in risk assessment and decision-making processes.
2. Real-time Monitoring: AI enables real-time monitoring of market conditions, enabling proactive risk management strategies and timely interventions.
3. Cost Efficiency: Automation of routine tasks through AI reduces operational costs associated with risk management and compliance.
4. Scalability: AI systems can handle large volumes of data and adapt to changing business environments, ensuring scalability without compromising performance.
Challenges and Considerations
While AI offers substantial benefits in risk management, it also presents challenges such as data privacy concerns, algorithmic bias, and regulatory compliance.
Financial institutions must implement robust governance frameworks to address these challenges and ensure ethical and responsible AI use.
The Future of AI in Risk Management
Looking ahead, the role of AI in risk management is expected to expand further with advancements in deep learning, quantum computing, and decentralized finance (DeFi).
These innovations will enable more sophisticated risk modeling, enhanced decision-making capabilities, and greater resilience against emerging risks.
Exploring Top AI Tools for Enhanced Risk Management in Finance
Financial analysts leverage various AI tools to enhance risk management practices. Here are some top AI tools used in risk management, along with details of each:
1. IBM Watson
Overview: IBM Watson is a comprehensive AI platform that integrates machine learning, natural language processing (NLP), and data analytics capabilities. It enables financial analysts to extract insights from structured and unstructured data, aiding in risk assessment and decision-making processes.
Key Features:
- Natural Language Processing (NLP): Analyzes text data from news, social media, and regulatory filings to assess market sentiment and identify emerging risks.
- Machine Learning Algorithms: Predictive analytics models help in forecasting market trends, credit risks, and other financial indicators.
- Risk Modeling: Creates sophisticated risk models based on historical data, enabling proactive risk management strategies.
Use Cases: IBM Watson is used for fraud detection, credit risk assessment, portfolio optimization, and real-time market monitoring.
2. QuantConnect
Overview: QuantConnect is a cloud-based algorithmic trading platform that utilizes AI and machine learning to develop and backtest trading strategies. It caters specifically to financial analysts and quantitative traders looking to automate trading decisions while managing risks effectively.
Key Features:
- Algorithm Development: Allows financial analysts to develop and deploy custom trading algorithms using Python or C#.
- Backtesting: Simulates trading strategies against historical data to assess performance and risk metrics.
- Integration with Brokerages: Enables seamless execution of trading strategies across multiple asset classes and markets.
Use Cases: QuantConnect is used for algorithmic trading, risk arbitrage, and volatility modeling to optimize portfolio returns while minimizing risks.
3. Ayasdi
Overview: Ayasdi is an AI platform that specializes in machine intelligence and automated model discovery. It is particularly useful for financial analysts seeking advanced analytics solutions for risk management and regulatory compliance.
Key Features:
- Topological Data Analysis (TDA): Uncovers hidden patterns and relationships within complex datasets to identify potential risks.
- Automated Model Discovery: Generates predictive models automatically from data, enhancing accuracy in risk assessment.
- Regulatory Compliance: Helps financial institutions comply with regulatory requirements through advanced analytics and reporting capabilities.
Use Cases: Ayasdi is used for fraud detection, anomaly detection, stress testing, and regulatory compliance in risk management.
4. Riskalyze
Overview: Riskalyze is a risk assessment and portfolio analysis platform that utilizes AI to quantify risk tolerance and optimize investment decisions. It is designed for financial advisors and analysts to align client portfolios with their risk preferences.
Key Features:
- Risk Assessment Tools: Utilizes AI algorithms to assess and quantify risk tolerance levels of investors.
- Portfolio Analytics: Analyzes portfolio performance metrics and identifies potential risks based on historical data.
- Scenario Analysis: Simulates different market scenarios to evaluate portfolio resilience and risk exposure.
Use Cases: Riskalyze is used by financial advisors for client risk profiling, portfolio stress testing, and personalized investment strategies.
5. SAS Risk Management
Overview: SAS Risk Management is an AI-powered software suite that provides comprehensive risk analytics and regulatory compliance solutions for financial institutions. It integrates AI and machine learning to enhance risk modeling and decision-making processes.
Key Features:
- Credit Risk Modeling: Uses AI to assess credit risk profiles of borrowers and predict default probabilities.
- Market Risk Analytics: Analyzes market data in real-time to identify fluctuations and potential risks to investment portfolios.
- Compliance Reporting: Automates regulatory reporting and ensures adherence to financial regulations using advanced analytics.
Use Cases: SAS Risk Management is used for credit risk assessment, market risk monitoring, stress testing, and regulatory compliance in financial institutions.
These AI tools represent a subset of the technologies empowering financial analysts in risk management.
By harnessing the capabilities of AI and machine learning, analysts can navigate complex financial landscapes with greater precision, make data-driven decisions, and mitigate risks effectively.
As AI continues to evolve, these tools are expected to play an increasingly crucial role in shaping the future of risk management within the banking and financial sector.
Master Affiliate Marketing with Wealthy Affiliate (WA)
“Join 10 Free Classes and Learn to Build a Profitable Online Business Through Affiliate Marketing, Tailored to Your Expertise.”
Step-by-Step Guidelines for Financial Analysts Using AI in Risk Management
1. Define Objectives and Scope
- Identify Goals: Clearly define the specific risk management objectives AI will address (e.g., credit risk assessment, fraud detection, market risk analysis).
- Scope: Determine the scope of AI application (e.g., which types of risks, which data sources).
2. Assess Data Availability and Quality
- Data Inventory: Conduct a thorough inventory of available data sources relevant to risk management.
- Data Quality: Evaluate data quality (accuracy, completeness, timeliness) and identify potential gaps or inconsistencies.
- Data Preprocessing: Clean, normalize, and prepare data for AI analysis, ensuring it meets quality standards.
3. Select Appropriate AI Tools and Algorithms
- Research AI Tools: Explore AI platforms and tools suitable for your specific risk management needs (e.g., IBM Watson, SAS Risk Management).
- Algorithm Selection: Choose algorithms based on the nature of risks (e.g., machine learning for predictive analytics, natural language processing for sentiment analysis).
- Customization: Collaborate with data scientists to customize algorithms or develop new ones as needed.
4. Develop and Train AI Models
- Model Development: Build AI models tailored to address identified risks and objectives.
- Training Data: Select relevant historical data for training AI models, ensuring representativeness and diversity.
- Validation: Validate models using testing datasets to assess accuracy, reliability, and performance metrics.
5. Integrate AI with Existing Systems
- System Compatibility: Ensure AI solutions integrate seamlessly with existing IT infrastructure and risk management systems.
- Data Integration: Establish pipelines for data ingestion, processing, and output integration.
- Security Measures: Implement robust security measures to protect sensitive data processed by AI systems.
6. Implement Governance and Compliance Frameworks
- Regulatory Compliance: Adhere to relevant regulations (e.g., GDPR, financial regulations) governing AI use in risk management.
- Ethical Considerations: Establish guidelines for ethical AI practices, ensuring fairness, transparency, and accountability.
- Governance Structure: Designate responsibilities for AI governance, including monitoring, auditing, and risk mitigation strategies.
7. Monitor Performance and Refinement
- Performance Metrics: Define key performance indicators (KPIs) to measure AI model effectiveness (e.g., accuracy, precision, recall).
- Continuous Monitoring: Monitor AI model performance in real-time, identifying anomalies or deviations from expected outcomes.
- Refinement: Continuously refine AI models based on new data, feedback, and changing risk factors.
8. Foster Collaboration and Decision Support
- Human-AI Collaboration: Encourage collaboration between financial analysts and AI systems, leveraging AI insights as decision support tools.
- Critical Thinking: Encourage critical thinking and validation of AI-generated insights to complement human judgment.
- Training and Development: Provide ongoing training and development opportunities for analysts to enhance AI proficiency and interpretation skills.
9. Evaluate and Iterate
- Feedback Loop: Establish a feedback loop to gather input from stakeholders and users regarding AI effectiveness and usability.
- Iterative Improvement: Use feedback to iterate and improve AI models, algorithms, and processes continuously.
10. Document and Communicate Results
- Documentation: Document AI methodologies, processes, and outcomes for transparency and future reference.
- Communication: Communicate findings, insights, and recommendations derived from AI analysis to stakeholders effectively.
By following these step-by-step guidelines, financial analysts can harness the power of AI to enhance risk management practices, make informed decisions, and navigate complex financial landscapes with greater agility and confidence.
Adopting AI in risk management is not just about technology implementation but also about strategic alignment, governance, and continuous improvement to achieve sustainable risk management outcomes.
Critical Success Factors in Integrating AI for Risk Management by Financial Analysts
When financial analysts integrate AI into risk management practices, several critical factors contribute to its effectiveness and success.
Here are key critical factors for financial analysts using AI in risk management:
1. Quality of Data
- Importance: AI algorithms rely heavily on data quality. Inaccurate or incomplete data can lead to flawed predictions and decisions.
- Considerations: Ensuring data integrity, consistency, and relevance is crucial. Data should be cleaned, normalized, and validated before AI analysis.
- Actions: Implement data governance frameworks, establish data quality standards, and employ robust data validation processes.
2. Algorithm Selection and Development
- Importance: Choosing appropriate algorithms is essential for accurate risk assessment and prediction.
- Considerations: Different types of risks may require different algorithms (e.g., machine learning for predictive analytics, natural language processing for sentiment analysis).
- Actions: Financial analysts should collaborate with data scientists to select and develop algorithms tailored to specific risk management objectives.
3. Interpretability and Explainability
- Importance: Understanding how AI models reach decisions is crucial for trust and regulatory compliance.
- Considerations: Complex AI models may be difficult to interpret. Clear explanations of model outputs are necessary for stakeholders.
- Actions: Use interpretable AI techniques where possible. Document and communicate model assumptions, inputs, and outputs transparently.
4. Integration with Existing Systems
- Importance: Seamless integration of AI tools with existing IT infrastructure and risk management systems ensures operational efficiency.
- Considerations: Compatibility, scalability, and security of AI solutions must align with organizational goals and IT policies.
- Actions: Conduct thorough system assessments, pilot projects, and collaborate with IT teams to integrate AI tools effectively.
5. Risk Governance and Compliance
- Importance: AI in risk management must adhere to regulatory guidelines and organizational policies.
- Considerations: Compliance with data privacy laws (e.g., GDPR, CCPA), financial regulations (e.g., Basel III), and internal risk management protocols.
- Actions: Establish governance frameworks for AI usage. Conduct regular audits, ensure ethical AI practices, and maintain regulatory compliance.
6. Continuous Learning and Adaptation
- Importance: Financial markets and risks evolve rapidly. AI models must adapt to changing conditions to remain effective.
- Considerations: Implement mechanisms for continuous learning and model refinement based on new data and feedback.
- Actions: Monitor model performance, update algorithms regularly, and invest in ongoing training for analysts and AI systems.
7. Human Oversight and Decision Support
- Importance: While AI enhances decision-making, human judgment remains indispensable in complex scenarios.
- Considerations: Financial analysts should use AI outputs as decision support tools rather than relying solely on automated recommendations.
- Actions: Foster collaboration between AI systems and human experts. Encourage critical thinking and validation of AI-generated insights.
Effective utilization of AI in risk management by financial analysts hinges on these critical factors.
By addressing data quality, algorithm selection, interpretability, system integration, governance, continuous learning, and human oversight, analysts can harness AI’s transformative potential to enhance risk assessment, decision-making, and overall organizational resilience in the face of dynamic financial environments.
AI has transformed risk management in the banking sector by empowering financial analysts to enhance decision-making, mitigate risks, and optimize operational efficiency.
By applying machine learning algorithms and predictive analytics, AI processes vast datasets quickly, identifying patterns that inform proactive risk management strategies. Key applications include predictive analytics for anticipating market trends, natural language processing for real-time insights from unstructured data, and algorithmic trading for dynamic portfolio optimization.
AI also improves accuracy and efficiency, enables real-time monitoring, and reduces operational costs. However, challenges such as data privacy, algorithmic bias, and regulatory compliance require robust governance frameworks.
Looking forward, advancements in AI promise to refine risk modeling further, shaping a resilient financial landscape.
Note: Click here to learn more on “How To Use AI in Your Business“.