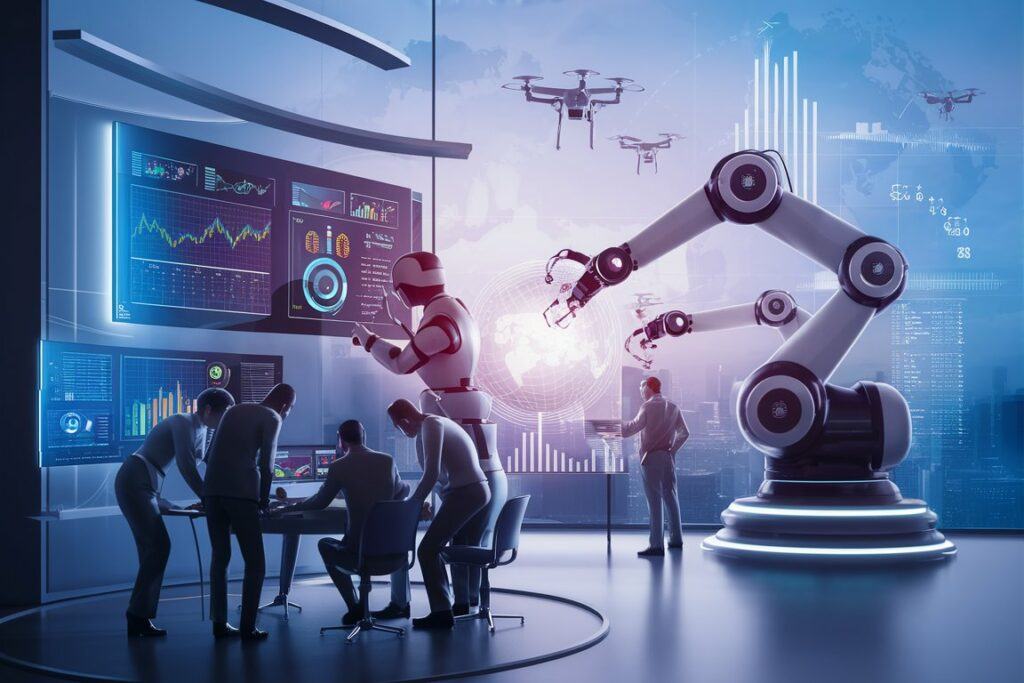
In the dynamic and fast-paced world of investment banking, the need for accurate, data-driven decision-making is paramount.
The incorporation of Artificial Intelligence (AI) into portfolio management has revolutionized the industry, enabling investment bankers to optimize asset allocation, mitigate risks, and enhance overall portfolio performance.
“Optimize Portfolios with AI Insights”
This article explores how AI is transforming portfolio management and why it is becoming an indispensable tool for investment bankers.
AI’s Role in Investment Bankers’ Portfolio Management
The Role of AI in Portfolio Management
AI, with its ability to process vast amounts of data and identify patterns that humans might overlook, has become a game-changer in portfolio management. Here’s how investment bankers are leveraging AI:
- Data Analysis and Predictive Analytics
- Data Integration: Investment bankers utilize AI to integrate data from various sources, including market trends, economic indicators, and financial statements. This holistic view enables more informed decision-making.
- Predictive Modeling: AI algorithms analyze historical data to predict future market movements. This helps in identifying potential investment opportunities and risks, allowing for proactive management.
- Algorithmic Trading
- High-Frequency Trading (HFT): AI-driven algorithms execute trades at high speeds and volumes, capitalizing on minor price discrepancies in the market. This enhances liquidity and can lead to significant profits.
- Market Sentiment Analysis: AI tools analyze social media, news articles, and other text data to gauge market sentiment. This real-time insight helps investment bankers adjust their trading strategies accordingly.
- Risk Management
- Risk Assessment Models: AI models assess the risk profile of portfolios by analyzing various risk factors, such as market volatility, credit risk, and geopolitical events. This ensures that portfolios are balanced and aligned with the investor’s risk tolerance.
- Stress Testing: AI simulates different market scenarios to test the resilience of portfolios. This helps in identifying vulnerabilities and making necessary adjustments to mitigate potential losses.
- Personalized Investment Strategies
- Client Profiling: AI analyzes clients’ financial goals, risk tolerance, and investment preferences to create personalized investment strategies. This ensures that each client’s portfolio is tailored to their specific needs.
- Robo-Advisors: AI-powered robo-advisors provide automated, algorithm-driven financial planning services. They offer investment advice and manage portfolios with minimal human intervention, making investment management accessible to a broader audience.
- Portfolio Optimization
- Asset Allocation: AI optimizes asset allocation by analyzing the performance of various asset classes and their correlations. This helps in diversifying portfolios to maximize returns and minimize risks.
- Rebalancing: AI continuously monitors portfolios and automatically rebalances them to maintain the desired asset allocation. This ensures that portfolios remain aligned with the investor’s goals and market conditions.
Benefits of AI in Portfolio Management
The integration of AI in portfolio management offers numerous benefits, making it an invaluable tool for investment bankers:
- Enhanced Efficiency: AI automates various processes, reducing the time and effort required for portfolio management. This allows investment bankers to focus on strategic decision-making.
- Improved Accuracy: AI algorithms analyze data with high precision, reducing the likelihood of human errors. This leads to more accurate predictions and better investment decisions.
- Real-Time Insights: AI provides real-time analysis and insights, enabling investment bankers to respond swiftly to market changes. This agility is crucial in the fast-paced world of investment banking.
- Scalability: AI systems can handle large volumes of data and transactions, making them suitable for managing complex portfolios. This scalability is essential for catering to diverse client needs and expanding business operations.
Challenges and Considerations
While AI offers significant advantages, its integration into portfolio management also presents challenges that investment bankers must address:
- Data Quality: The effectiveness of AI models depends on the quality of data. Investment bankers must ensure that they have access to accurate and reliable data to avoid erroneous predictions and decisions.
- Regulatory Compliance: AI-driven portfolio management must comply with regulatory standards. Investment bankers need to ensure that their AI systems adhere to these regulations to avoid legal and financial repercussions.
- Transparency and Explainability: AI models can be complex and difficult to interpret. Investment bankers must ensure that their AI systems are transparent and provide explanations for their decisions to maintain client trust and meet regulatory requirements.
- Cybersecurity: The use of AI in portfolio management involves handling sensitive financial data. Investment bankers must implement robust cybersecurity measures to protect this data from cyber threats.
Future Trends
The use of AI in portfolio management is continuously evolving, with several emerging trends set to shape the future of the industry:
- Advanced Machine Learning: Investment bankers are increasingly adopting advanced machine learning techniques, such as deep learning and reinforcement learning, to enhance their AI models. These techniques offer more sophisticated analysis and prediction capabilities.
- Integration with Blockchain: The integration of AI with blockchain technology promises to enhance the transparency, security, and efficiency of portfolio management. This combination can streamline processes such as asset tracking, transaction verification, and compliance reporting.
- AI-Driven ESG Investing: Environmental, Social, and Governance (ESG) investing is gaining popularity. AI can analyze ESG data to help investment bankers identify sustainable and socially responsible investment opportunities.
- Natural Language Processing (NLP): NLP enables AI systems to understand and analyze human language. Investment bankers can leverage NLP to gain insights from unstructured data, such as financial reports, news articles, and social media posts.
How Investment Bankers Utilize AI in Portfolio Management: Top 5 AI Tools
Investment bankers are increasingly turning to AI tools to enhance their portfolio management strategies. Here are the top five AI tools and products that are making a significant impact in the industry:
1. Aladdin by BlackRock
Highlights:
- Comprehensive Risk Management: Aladdin integrates sophisticated risk analytics, allowing investment bankers to assess and mitigate portfolio risks effectively.
- Predictive Analytics: Utilizes machine learning to provide predictive insights on market trends and asset performance.
- Operational Efficiency: Streamlines portfolio management operations with advanced automation and data integration capabilities.
2. Kensho by S&P Global
Highlights:
- Data Analysis and Visualization: Kensho’s AI-driven analytics help investment bankers make sense of vast amounts of financial data through intuitive visualizations.
- Market Intelligence: Provides real-time analysis of market movements, news, and events to inform investment decisions.
- Natural Language Processing (NLP): Analyzes text data from various sources to gauge market sentiment and potential impacts on portfolios.
3. SymphonyAI Sensa
Highlights:
- Fraud Detection and Compliance: Uses AI to detect anomalies and potential fraud in transactions, ensuring regulatory compliance.
- Risk Assessment: Offers advanced risk analytics and scenario modeling to help manage portfolio risks.
- Client Insights: Leverages AI to gain deeper insights into client behaviors and preferences, enabling personalized investment strategies.
4. AlphaSense
Highlights:
- AI-Powered Search Engine: Enables investment bankers to quickly find relevant information from a vast array of financial documents and market data.
- Sentiment Analysis: Uses NLP to analyze sentiment from earnings calls, news, and reports, helping bankers understand market and company outlooks.
- Real-Time Data: Provides up-to-date information on market trends, helping to inform timely investment decisions.
5. Bloomberg Terminal
Highlights:
- Comprehensive Market Data: Offers extensive financial data, news, and analytics for in-depth market research and analysis.
- Machine Learning Models: Incorporates AI-driven models to forecast market trends and asset performance.
- Portfolio Analytics: Provides tools for portfolio optimization, risk assessment, and scenario analysis, enhancing overall portfolio management efficiency.
These AI tools are transforming how investment bankers manage portfolios, offering advanced analytics, predictive insights, and operational efficiencies that are critical in today’s fast-paced financial markets. By leveraging these technologies, investment bankers can stay ahead of market trends, make more informed decisions, and deliver better outcomes for their clients.
Join Free 10 Classes by Wealthy Affiliate (WA)
Learn how to build a profitable and sustainable online business through affiliate marketing tailored to your expertise.
How Investment Bankers Utilize AI in Portfolio Management: Step-by-Step Guidelines
Incorporating AI into portfolio management can significantly enhance the effectiveness and efficiency of investment strategies. Below are step-by-step guidelines on how investment bankers can utilize AI in portfolio management:
Step 1: Data Collection and Integration
Actions:
- Identify Data Sources: Determine relevant data sources, such as financial statements, market trends, economic indicators, and news articles.
- Automate Data Collection: Use AI tools to automate the collection of data from various sources to ensure real-time updates.
- Data Cleaning: Ensure data is clean and free of errors or inconsistencies to improve the accuracy of AI models.
- Integrate Data: Consolidate data from different sources into a unified database for comprehensive analysis.
Step 2: Data Analysis and Predictive Modeling
Actions:
- Select AI Tools: Choose AI tools that specialize in data analysis and predictive modeling.
- Historical Data Analysis: Use AI to analyze historical data to identify patterns and trends.
- Predictive Analytics: Develop predictive models to forecast future market movements and asset performance.
- Scenario Analysis: Simulate different market scenarios to understand potential impacts on the portfolio.
Step 3: Algorithmic Trading and Execution
Actions:
- Develop Trading Algorithms: Create AI-driven trading algorithms based on predictive models and market conditions.
- Backtesting: Test the algorithms on historical data to ensure their effectiveness and reliability.
- Real-Time Execution: Implement the algorithms for real-time trading to capitalize on market opportunities.
- Monitor Performance: Continuously monitor the performance of the algorithms and make adjustments as needed.
Step 4: Risk Management
Actions:
- Risk Assessment Models: Utilize AI models to assess the risk profile of the portfolio by analyzing various risk factors.
- Stress Testing: Conduct stress tests using AI to evaluate how the portfolio would perform under different adverse conditions.
- Risk Mitigation Strategies: Develop and implement strategies to mitigate identified risks, such as diversification or hedging.
- Continuous Monitoring: Use AI to continuously monitor the portfolio for emerging risks and adjust strategies accordingly.
Step 5: Portfolio Optimization and Rebalancing
Actions:
- Asset Allocation: Use AI to optimize asset allocation by analyzing the performance and correlation of different asset classes.
- Optimization Models: Implement AI-driven optimization models to maximize returns and minimize risks.
- Rebalancing: Set up AI systems to automatically rebalance the portfolio based on predefined criteria and market conditions.
- Performance Tracking: Continuously track portfolio performance and make necessary adjustments to maintain alignment with investment goals.
Step 6: Personalized Investment Strategies
Actions:
- Client Profiling: Use AI to analyze clients’ financial goals, risk tolerance, and investment preferences.
- Customized Portfolios: Develop personalized investment strategies tailored to each client’s unique profile.
- Robo-Advisors: Implement AI-powered robo-advisors to provide automated financial planning and investment management services.
- Client Reporting: Use AI to generate customized reports for clients, providing insights into portfolio performance and recommendations.
Step 7: Compliance and Security
Actions:
- Regulatory Compliance: Ensure AI systems comply with regulatory standards and guidelines.
- Transparent Reporting: Maintain transparency in AI-driven decisions and provide explanations to clients and regulators.
- Cybersecurity Measures: Implement robust cybersecurity protocols to protect sensitive financial data from cyber threats.
- Audit and Monitoring: Regularly audit AI systems and monitor their performance to ensure compliance and security.
By following these step-by-step guidelines, investment bankers can effectively integrate AI into portfolio management, enhancing their ability to make informed decisions, manage risks, and optimize portfolio performance.
In conclusion, the integration of Artificial Intelligence (AI) into portfolio management represents a pivotal advancement in the field of investment banking. AI empowers bankers with unprecedented capabilities to analyze vast datasets, predict market trends, and execute trades with precision, thereby optimizing asset allocation and enhancing portfolio performance.
Beyond operational efficiencies and improved accuracy, AI-driven tools like predictive analytics, algorithmic trading, and personalized investment strategies offer substantial benefits, enabling bankers to navigate complexities and deliver superior outcomes for clients.
While challenges such as data quality and regulatory compliance persist, the ongoing evolution of AI technologies promises continued innovation and transformation in portfolio management, cementing AI’s role as a cornerstone in modern investment strategies.
Note: Click here to learn more about “How to Use AI in Your Business.”